One of the world’s most vibrant growth industries, projected to reach a $150 billion valuation by 2026, artificial intelligence (AI) stands on the front-line of technological innovation. A prominent example of AI’s increasing prominence is the healthcare industry. AI services has genuinely revolutionized modern healthcare from streamlining diagnoses and treatment recommendations to automating disease detection and segmentation.
Streamlining and Improving Diagnostic Processes
Given the potentially fatal consequences of human error resulting from faulty diagnoses – e.g., incomplete medical histories case overloads—improving diagnostic practices is one of AI’s most essential implementations within the healthcare industry. Notable approaches include:
- Using supervised machine learning to predict the most successful treatment protocol(s) according to a patient’s attributes and treatment context
- Employing neural networks to predict whether or not a patient will acquire a disease
- Utilizing deep learning applications to recognize cancerous masses/lesions in radiology scans.
Moreover, Natural Language Processing applications may analyze unstructured clinical patients’ notes, prepare reports, and transcribe patient interactions. All this being said, the most intriguing and potentially impactful approach is the implementation of deep learning-based technology solutions for automatic disease diagnosis.
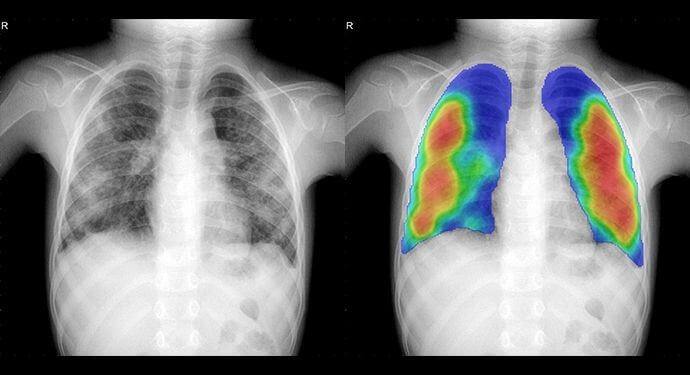
Deep-learning Based Disease Detection and Segmentation
Implementing clinical decision support algorithms for medical imaging is challenged by issues of reliability and interpretability. However, lots of promising research is being done to rectify this issue. For example, Kermany et al. (2018) present a deep-learning-based diagnostic tool using transfer learning to help screen patients with treatable blinding retinal diseases.
Impressively, this approach requires significantly fewer data to train a neural network than conventional methods. Upon testing, their model’s performance is comparable to that of human experts, while also providing more transparent and interpretable diagnoses by highlighting the regions recognized by the neural network. Broadly speaking, this approach may enable expedited diagnosis and referral of many treatable conditions, leading to earlier treatment, and ultimately improving clinical outcomes.
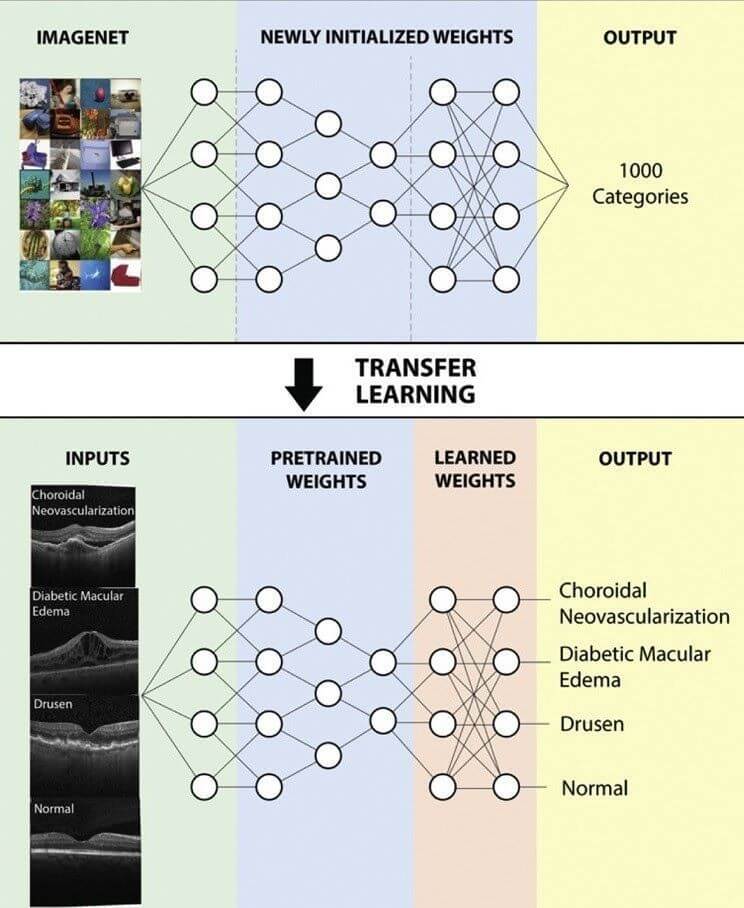
In another subfield, Coudray et al. (2019) investigate the efficacy of deep learning models in the visual inspection of histopathology slides—a standard method for pathologists to assess stage, type, and subtype of lung tumors. Comparing the performance of their deep
convolutional neural network on whole-slide images against pathologists, they conclude that deep learning models can help pathologists detect cancer subtypes or gene mutations.
Notably, their approach can be applied to any cancer type.
Although AI services shows the potential to revolutionize the disease diagnostic process via medical imaging, its efficacy in disease detection via medical text data, sensor fusion and other forms of medical examination—e.g., diabetes, hyperlipidemia, etc.—leaves much to be desired.
Traditional methods for automatic diagnoses focus on patient descriptions, researchers extracting features, and coding them to form eigenvectors, classified via classification
algorithms. Many problems arise when said methods are applied to medical text data, with manual feature extraction and a lack of relevant raw data bringing into question the results’ objective accuracy. Some studies suggest expanding learning algorithms as a viable solution to the latter issue—i.e., supplementing the original, lacking data with comprehensive data to cover any raw data shortages. Zhang et al. (2019) employ an expanding learning algorithm in a deep-learning-based automatic diagnostic system, which has produced promising results.
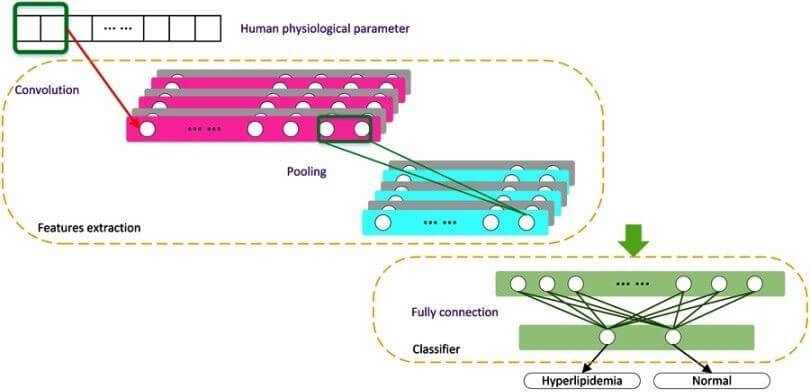
It diagnoses hyperlipidemia based on human hematologic and urinary parameters, automatically extracting all available information from the raw data without any human involvement. With accurate performance and a marked reduction in labor costs, its implementation ultimately improves clinical diagnostic efficiency. Moreover, their core model may be generalized to apply to a “wider range of medical text data to achieve assistant clinical diagnosis.”
Though none of these approaches are without fault, their research outcomes encourage examples of AI Services positive, long term impact upon healthcare.